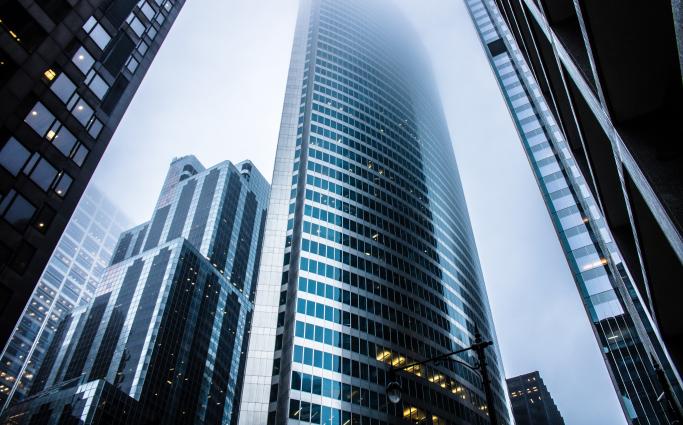
Key Takeaways
The unglamorous, time-consuming work of data cleansing and feature engineering is essential to success, requiring “domain expertise” (knowledge of both fundamental equity analysis and computer science).
There is no magic algorithm. We’ve found that a dynamic ecosystem of machine learning models are better suited to capturing the complexities of financial investing.
It turns out an important ingredient in AI-driven investing is … people! Human-plus-machine is better than either alone.
Domain expertise is essential
Investing with artificial intelligence (AI) isn’t just about data and algorithms—it is deeply intertwined with human expertise in financial analysis, economics and even geopolitics. This domain expertise informs every step of the investment process, especially when curating data and designing features.
CFAs and PhDs working together
Financial data is non-stationary by nature and can be messy, inconsistent and noisy. You can’t just apply deep learning to raw data and expect reliable results. Consider how chartered financial analysts (CFAs1) carefully vet and standardize quarterly results when entering them into a spreadsheet. Adding AI doesn’t change that.
Scrubbing and transforming data may be unglamorous grunt work, but it’s the unsung hero of AI-driven investing. Domain experts are aware of financial data quality issues (such as inaccuracies, missing values, inconsistencies or outdated information) and know how to preprocess data effectively for machine learning (ML) models. Our CFAs and computer science PhDs collaborate to design models that meticulously curate thousands of inputs per company across macro, sentiment, technical, fundamental and alternative data sets.
Feature engineering
In AI-driven investing, features are highly engineered financial ratios that reduce the dimensionality of data (from over 10,000 to about 250) and translate financial expertise into representations that capture the nuances of stock dynamics. While off-the-shelf AI feature libraries can be a starting point, we have found they are far from sufficient and lack the proprietary insight necessary in the competitive world of finance.
This is where domain expertise comes into play—intensive, time-consuming work dedicated to tailoring data inputs and crafting knowledge-informed features. This process is similar to how portfolio managers distill company data down into their preferred set of traditional analytic ratios, such as price to book and price to sales, or more subjective factors that contribute to a strong company, such as board diversity. With AI, it is possible to incorporate hundreds of features across a wider range than any human analyst could manage for even a single stock.
Feature engineering isn’t easy, but it’s critical for extracting relevant information, reducing noise and revealing deep, repeatable patterns in the stock universe.
There’s no one magic algo to rule them all
One of the most pervasive misconceptions about AI in the realm of finance is that there is a single all-encompassing algorithm that will effortlessly solve the complex puzzle of investing. Maybe when today’s toddlers are running the world, machines will have solved investing and all the other riddles of human existence. Until then, we must think more realistically.
While there is more than one way to investment heaven (as our own Gareth Shepherd likes to say), we’ve found that when applying AI to fundamental investing, a dynamic ensemble of ML models is most effective. We use 26 distinct models (we call them “virtual analysts”), split between long and short, each tuned to different time horizons and goals (or “objective functions” in machine learning lingo). Through a process of competition and cooperation, our models provide stock recommendations, with the highest-conviction ideas winning the largest allocations.
Human plus machine is better than either alone
AI represents a paradigm-altering moment in history, and it’s reshaping how managers navigate the intricacies of investing. But the notion that AI is inherently better than humans oversimplifies the complex interplay between technology and human know-how.
Rather than competing, the two can complement each other to tackle challenges more effectively. It’s not a matter of superiority but of collaboration to harness the best of both worlds. By pairing the domain expertise of humans with AI’s ability to quickly identify patterns and act without emotion, we have a new, powerful way of investing.
Change, before you have to
It’s human nature to think in linear terms— that the next decade will produce about the same level of innovation as the last decade. But technology doesn’t evolve at a steady pace. It accelerates. What might be considered cutting edge today could easily find itself obsolete tomorrow. The winners never stop innovating.
Dedication to continuous improvement shouldn’t be confined to incremental changes (though that is part of it). Envision what the future may hold, then harness emerging technologies to realize it. The R&D done today serves as the foundation for what can be achieved a year from now, and the year after that. In a short time, the future may exceed the furthest reaches of human imagination.
The resounding lesson: For managers to remain relevant and competitive in this ever-evolving field, they must pursue unceasing progress. The journey doesn’t culminate with a single achievement; it extends into a continuous cycle of advancement, where innovation is both the means and the end.
Bottom line Success in finance isn’t solely contingent on AI adoption. It’s an intricate dance that calls for a deep understanding of nuances, an unwavering respect for fundamentals, and an enduring commitment to continuous improvement. |