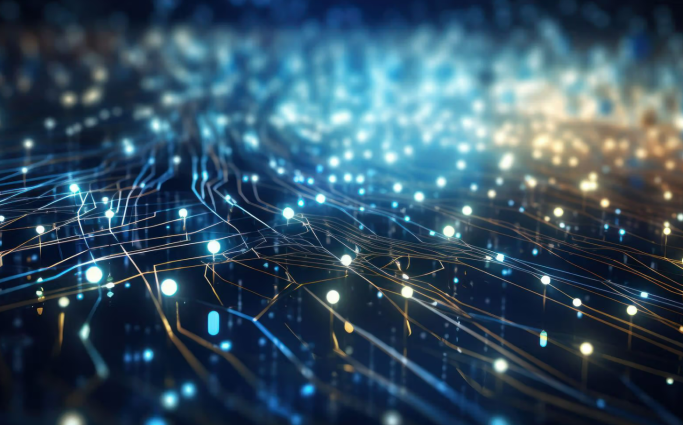
The future of asset management is rapidly approaching—one in which the strengths of humans and machines are integrated to potentially make faster, better decisions. This guide is for allocators looking to understand how AI/ML models operate in an investment context, which ones are most effective in different situations, and how Voya approaches the complexities of practical implementation.
Executive summary
As with any technological leap, the integration of machine-driven decisionmaking capabilities into investment strategies demands a heightened level of due diligence. As a former equity manager researcher, I’ve been through the process and I understand the daunting task that asset allocators face. Shorter live track records are inevitable when dealing with new technologies (and you’ll never see a “bad” backtest). It’s important to know how AI models function within an investment context and which ones are effective for different scenarios. And then there’s the unavoidable issue of how to compare AI-driven strategies against established quantitative and fundamental strategies.
This guide is for investors seeking a better framework for assessing the merits of various approaches in this rapidly evolving field. We have structured the discussion around three key topics:
Applying AI models to investing
Effective investment strategies require a balanced use of human expertise and rational analysis alongside AI data-processing and problem-solving capabilities. Understanding the pros and cons of each is critical to identifying new ways for humans and machines to work together in investing.
Designing an AI investment framework
In a world of messy and limited information, successful AI integration starts with human domain experts curating data—helping to ensure diverse, high-value inputs—and providing some ground rules. Then it’s a matter of matching the requirements and parameters of a specific investment task with the right combination of AI (and human) tools.
Defining best practices
Voya Machine Intelligence uses a “neuro-symbolic” process that relies on human skills for data preparation and feature engineering, and it taps machine capabilities for pattern recognition and conviction rankings. Grounded in the team’s 10+ years of experience,1 this framework has led to unique investment ideas and alpha generation in a cost-effective manner, thus raising the bar for both fundamental and quantitative managers.
Glossary of terms
Artificial intelligence (AI): The capability of engineered or machine-based systems to perform functions that are normally associated with human intelligence such as reasoning, learning, and self-improvement.
Machine learning (ML): A subset of AI that involves training algorithms to learn from and generate predictions or decisions based on previous data, without being explicitly programmed for each specific task.
Machine learning models: Algorithms created through machine learning that process input data and make data-driven predictions or decisions from unseen data, based on previous inputs.
Natural language processing (NLP): A subset of artificial intelligence that allows computers to understand, interpret, and generate human language in a way that is useful and meaningful.
Generative AI (gen AI): A type of artificial intelligence that is used to create new content, ranging from text to images and music, by learning from a large pool of existing data and generating outputs that mimic the original data.
Machine intelligence: The capability of a machine to imitate intelligent human behavior, encompassing many forms of AI, including but not limited to machine learning, deep learning, natural language processing and generative AI.
Voya Machine Intelligence (VMI): The VMI team leverages machine learning models for fundamental analysis. It does not utilize generative AI in the stock selection process.
Virtual analyst: A machine learning algorithm(s) that analyzes data and looks for patterns within the data.
Human-supervised AI: AI systems operating under the guidance of humans. The VMI team employs machine learning models trained on features created by humans with domain expertise.
A note about risk
The principal risks are generally those attributable to stock investing. Holdings are subject to market, issuer and other risks, and their values may fluctuate. Market risk is the risk that securities may decline in value due to factors affecting the securities markets or particular industries. Issuer risk is the risk that the value of a security may decline for reasons specific to the issuer, such as changes in its financial condition.
Investment model: A manager’s proprietary model may not adequately allow for existing or unforeseen market factors or the interplay between such factors, and even a model that performs in accordance with the manager’s intentions may underperform other investment strategies or result in greater losses than other strategies. The proprietary models used by a manager to evaluate securities or securities markets are based on the manager’s understanding of the interplay of market factors and do not assure successful investment. The markets, or the prices of individual securities, may be affected by factors not foreseen in developing the models. Strategies that are actively managed, in whole or in part, according to a quantitative investment model, including models using artificial intelligence to select securities, can perform differently from the market as a whole based on the investment model and the factors used in the analysis, the weight placed on each factor, and changes from the factors’ historical trends. Mistakes in the construction or implementation of the investment models (including, for example, data problems and/or software issues) may create errors or limitations that might go undetected or be discovered only after the errors or limitations have negatively impacted performance. There is no guarantee that the use of these investment models will result in effective investment decisions for the Strategy.
Artificial intelligence (AI): AI—including natural language processing, machine learning and other forms of AI—may pose inherent risks, including but not limited to: issues with data privacy, intellectual property, consumer protection, and anti-discrimination laws; ethics and transparency concerns; information security issues; the potential for unfair bias and discrimination; quality and accuracy of inputs and outputs; technical failures and potential misuse. Reliance on information produced using AI-based technology and tools should factor in these risks. When using a quantitative model, including those that utilize AI, as part of an investment strategy, please note data imprecision, software or other technology malfunctions, programming inaccuracies and similar circumstances may impair the performance of these systems, which may negatively affect performance. Furthermore, there can be no assurance that the quantitative models used in managing a strategy will perform as anticipated or enable the strategy to achieve its objective. The Voya Machine Intelligence (VMI) team employs a proprietary machine learning approach to identify and exploit persistent patterns in company data. The approach leverages (non-linear) machine learning (“ML”) models for fundamental analysis. The ML models employed do not utilize generative AI algorithms.